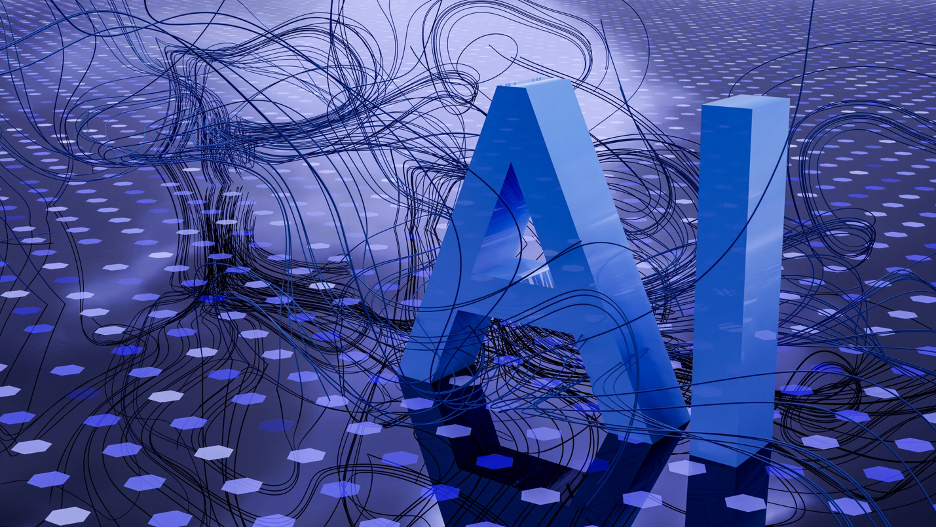
In recent years, artificial intelligence (AI) technology has advanced rapidly, especially with the increasingly widespread application of large models such as GPT-4, Claude, Gemini, WuDao 2.0, ERNIE, and PanGu-α. From natural language processing to image recognition and autonomous driving, AI large models have demonstrated immense potential across various fields. However, despite their outstanding capabilities in handling complex tasks, these AI large models are not without flaws. Nevertheless, they continue to play crucial roles in various professional domains, strongly driving industry development and innovation.
The Extraordinary Capabilities of AI Large Models
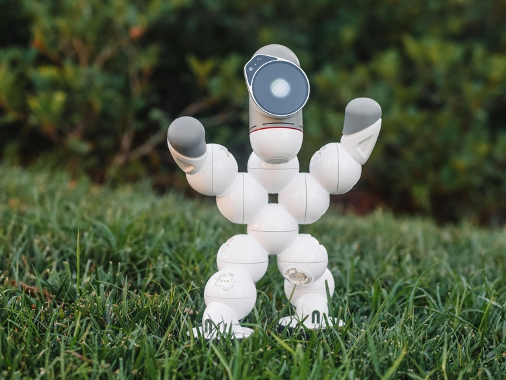
Powerful Processing Capability and Efficiency
AI large models possess robust data processing capabilities, enabling them to analyze and handle vast amounts of data in a short period. For example, in the financial sector, large models can quickly analyze global market data, providing real-time market predictions and investment advice. In the medical field, AI can assist doctors in precise diagnosis and personalized treatment by analyzing a large number of medical images and patient data.
For instance, a medical institution using AI models to analyze patients’ X-rays has significantly improved the accuracy and speed of early lung cancer diagnosis, saving many lives.
High Degree of Automation and Intelligence
AI large models can automatically execute complex tasks, reducing human intervention and errors. In the legal field, AI can automatically review massive legal documents, extract key information, and improve the efficiency and accuracy of legal work. For example, a law firm uses AI tools to quickly organize and analyze thousands of pages of contract documents, saving substantial time and human resources.
In the education sector, intelligent tutoring systems can adjust teaching content and pace based on students’ learning conditions, providing a personalized learning experience. Some schools are already using AI-assisted teaching to help students better understand and master knowledge.
Continuous Learning and Self-Optimization
AI large models have the ability to continuously learn and self-optimize, improving their performance and accuracy through ongoing training and feedback. For example, in the field of natural language processing, AI models can enhance their understanding of different languages and contexts by continuously learning new language data.
In autonomous driving, AI can optimize driving decisions and improve road safety by continuously accumulating driving data. For example, Tesla’s autopilot system improves its driving performance and safety through the accumulation and learning of extensive driving data.
Multi-Modal Processing Capability
AI large models are not limited to processing a single type of data; they can handle multiple data types simultaneously, including text, images, audio, and video. This multi-modal processing capability makes AI more flexible and efficient in many application scenarios. For example, an intelligent home system uses AI models to process voice commands and video surveillance data simultaneously, achieving smarter home management. Users can control appliances through voice commands, while the AI system ensures home safety through video surveillance.
Natural Language Understanding and Generation
AI large models perform exceptionally in natural language processing, capable of understanding and generating natural language. This makes them particularly outstanding in fields such as customer service, translation, and content creation. For example, some companies use AI customer service systems to handle customer inquiries, significantly improving response speed and accuracy. Additionally, AI models can automatically generate articles, reports, and advertising copy, enhancing the efficiency and quality of content creation.
High Scalability
AI large models possess high scalability and can adapt to tasks of different scales and complexities. This allows them to operate effectively in various scenarios, from small applications to large systems. For example, a retail company uses AI models to analyze customer purchasing behavior, thereby optimizing inventory management and marketing strategies, enhancing overall operational efficiency.
Cross-Domain Application Capability
AI large models can be widely applied across multiple fields, demonstrating their adaptability across domains. This allows them to find effective application scenarios in different industries. For instance, AI large models have been widely used in medical, financial, educational, legal, and other fields, promoting innovation and development in various industries.
Limitations of Large Model Technology
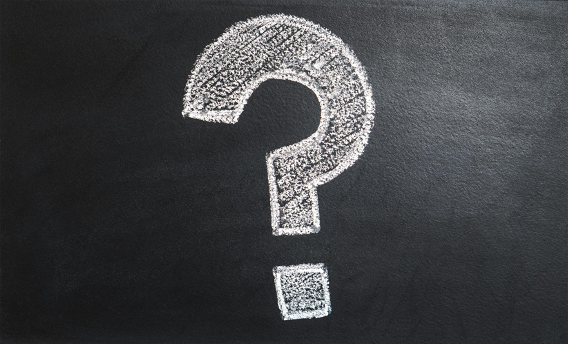
Data Bias and Fairness Issues
The training of large models relies on vast amounts of data, but these data often contain biases. For example, language models may reflect gender, racial, and other biases present in the training data, leading to unfair output results. In the medical field, if training data mainly come from certain groups, the model may be inaccurate in diagnosing other groups. For example, if an AI diagnostic system is primarily trained on medical data from white people in Europe and America, it may lack accuracy when diagnosing Asians or Africans.
Lack of Interpretability and Transparency
Many large models, especially deep learning models, are “black box” models, making it difficult to explain their internal workings and decision-making processes. This is a significant challenge for fields requiring high interpretability, such as financial decision-making and legal consulting. For instance, a financial institution uses an AI model for investment decisions, but due to the model’s opaque decision-making process, investment managers find it difficult to fully trust and rely on these decisions, affecting its application effectiveness.
Privacy and Security Issues
When handling sensitive data, AI large models pose risks to privacy and security. For example, in the medical field, patients’ private data may be leaked during data transmission and processing; in the financial sector, users’ financial data may be improperly used or attacked. Some medical institutions, due to inadequate data protection measures when using AI diagnostic systems, have led to the leakage of patients’ private data, triggering serious legal and ethical issues.
Hallucination Output Problem
AI large models sometimes generate inaccurate or even false information, a phenomenon known as the “hallucination output” problem. The reasons include not only the model’s method of predicting the next word based on context when generating text but also other factors such as the quality of training data, the model’s architecture, and data processing methods. If the training data contain erroneous information or biases, the model may reflect these issues when generating content. This poses risks for professional fields that rely on accurate information, such as healthcare and law.
When users utilize these AI large models, they may find it difficult to judge the correctness of the output, especially in the absence of relevant professional knowledge. In such cases, users may be misled by incorrect information, leading to erroneous decisions. For example, in the medical field, an AI diagnostic tool might generate incorrect advice when diagnosing diseases due to biases or errors in the training data, potentially affecting doctors’ diagnostic decisions. To reduce this risk, users should rigorously verify the model’s output and cross-reference with other reliable information sources.
Technical and Professional Knowledge Requirements
Although AI large models have enormous application potential, their deployment and maintenance require highly specialized technical knowledge and experience. Many enterprises and organizations lack relevant technical capabilities, making it difficult to fully leverage AI’s advantages. Additionally, developing and maintaining AI models requires significant resource investment, which may pose a substantial burden on small and medium-sized enterprises.
Integration of Domain Knowledge and Adaptability
Although AI large models have demonstrated excellent capabilities in many fields, in some specific professional areas, the models need to further integrate deep domain knowledge. How to effectively incorporate domain experts’ knowledge into AI models to enhance their performance in professional tasks is an important research direction. Below are some possible solutions:
- Integration of Large Models and Specialized Small Models
In complex application scenarios, combining large models with specialized small models can improve performance. The large model handles general tasks, while the specialized small model focuses on specific domain tasks. This approach leverages each model’s strengths, enhancing overall system accuracy and efficiency.
Example: In the medical field, a general large language model can be combined with a specially trained medical diagnostic model. The large model handles preliminary analysis of patients’ basic information, while the specialized small model conducts in-depth diagnosis and offers advice for specific diseases. This integration improves diagnostic accuracy and reduces doctors’ workload.
- Secondary Training with Specialized Data
To enhance performance in specific fields, AI large models can undergo secondary training using domain-specific data for fine-tuning. This method allows the model to maintain its general capabilities while improving understanding in specific domains.
Example: In the legal field, large models can be retrained using legal documents, cases, and regulations to make them more suitable for legal tasks. This enables AI models to more accurately generate legal documents, conduct research, and analyze cases.
- Integration of Knowledge Graphs
Incorporating domain experts’ knowledge into AI models via knowledge graphs can significantly enhance understanding and reasoning abilities. Knowledge graphs structurally represent domain knowledge, helping models grasp complex professional concepts and relationships.
Example: In pharmaceuticals, knowledge graphs of drug chemical structures, pharmacological actions, and clinical trial data can be used with AI models. This allows AI to analyze and predict new drug potentials and conduct precise drug development and optimization.
- Continuous Learning and Feedback Mechanism
Establishing continuous learning and feedback mechanisms ensures AI models’ ongoing improvement in professional fields. Regularly updating training data and incorporating expert feedback optimizes model performance.
Example: In education, AI tutoring systems can adjust teaching content and methods based on student feedback and learning data, enhancing teaching effectiveness and satisfaction.
- Multi-Modal Data Integration
Combining various data types can provide comprehensive analysis and decision support. Integrating text, images, audio, and sensor data can significantly enhance performance.
Example: In intelligent manufacturing, sensor data from production processes can be combined with process documentation. AI models can analyze this data to optimize production, improving product quality and efficiency.
User Dependence and Trust Issues
Users’ dependence on AI systems and trust issues require attention. Over-reliance on AI may lead users to overlook their own judgment, making it difficult to detect and correct errors. Building user trust in AI systems and ensuring reliability in critical decisions is a significant challenge.
Challenges in Applying Large Models
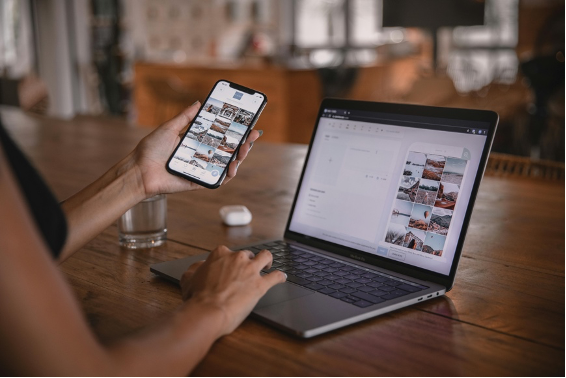
User Interface and Experience
- Complexity and Usability
Many AI models and tools have complex interfaces that are difficult for ordinary users to navigate. Data analysis tools or programming interfaces often require technical backgrounds, posing barriers for inexperienced users.
- Intuitiveness and User-Friendliness
Users need intuitive, easy-to-use interfaces. However, many AI applications lack user-friendly designs, leading to confusion and frustration.
Data Privacy and Security
- Data Privacy Concerns
AI models require vast data for training and operation, necessitating personal data from users. This raises concerns about privacy and security. For example, leakage of medical or financial information can have severe consequences.
- Data Protection
Users may lack the knowledge to secure data during transmission and storage, increasing leakage risks.
Reliability and Accuracy of Output
- Hallucination Output
AI models sometimes generate inaccurate or false information, known as “hallucination output.” Users may find it difficult to judge correctness, leading to erroneous decisions. For instance, AI-generated articles may mislead users with incorrect information.
- Interpretability and Transparency
AI decision-making processes often lack transparency, making it hard for users to understand outputs. This “black box” nature can reduce trust.
Technical and Professional Knowledge Requirements
- Technical Barriers
Many AI applications require programming skills and data analysis abilities. Users without these skills may struggle to utilize AI functions fully.
- Learning Curve
Even user-friendly tools have learning curves. Users must invest time and effort, which can be a significant barrier.
Future Prospects of AI in Medical, Legal, and Educational Fields
I. Medical Field
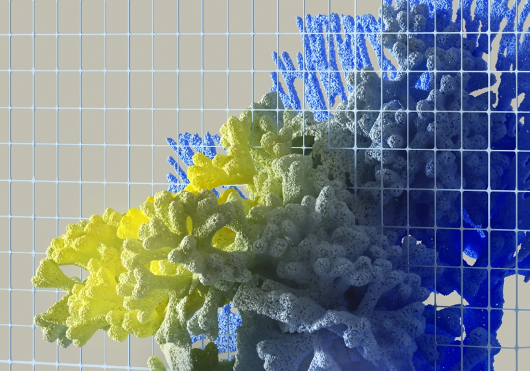
Precision Medicine and Personalized Treatment
AI models may integrate biological and medical big data to analyze patients’ genetic data, medical histories, and lifestyles, offering personalized treatment plans. For complex diseases, considering genetic subtypes and environmental differences enhances treatment efficacy. For example, analyzing cancer patients’ genetic data can predict drug responses, balancing efficacy and side effects for optimal treatment.
Early Disease Detection and Prediction
AI models have enormous potential in early disease detection. By analyzing medical images and health records—or using data from wearables like Apple Watch ECGs—AI can identify early disease signs, enabling timely interventions. For instance, AI can detect diabetic retinopathy early by analyzing retinal images, preventing blindness.
Health Management and Chronic Disease Monitoring
AI models play roles in health management and chronic disease monitoring by integrating daily health data like heart rate and blood glucose. They provide personalized advice, helping patients manage health effectively. A smart bracelet with AI can monitor heart rate changes in cardiac patients, providing real-time alerts.
Potential in the Pharmaceutical Industry
AI models show great promise in pharmaceuticals by analyzing chemical and biological data, accelerating drug discovery and development. AI can predict compounds’ therapeutic potentials, reducing time and costs. It also optimizes clinical trial designs, improving success rates. A pharmaceutical company used AI to discover a new drug molecule in months, expediting development.
Optimization of Medical Resources
AI models help optimize medical resource allocation and management, improving service efficiency. Analyzing patient flow and resource usage, AI predicts peak periods to arrange resources proactively, reducing wait times and enhancing hospital operations.
Telemedicine and Diagnosis
AI models enhance telemedicine by combining diagnostic tools with remote equipment. Doctors provide high-quality services remotely, using AI to analyze uploaded images and records. This improves accessibility and convenience in healthcare.
II. Legal Field
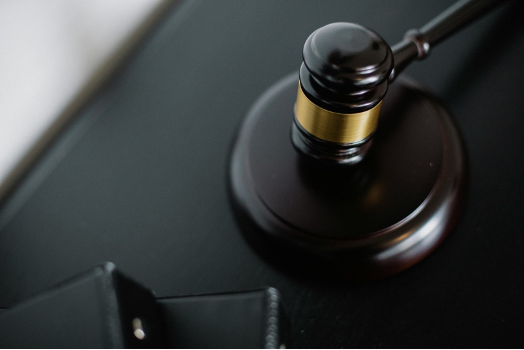
Automatic Generation of Legal Documents
AI models can automatically generate legal documents, boosting lawyers’ efficiency. For example, software like Lightyear AI Legal Assistant creates initial complaints, contracts, or agreements based on case information, reducing workload.
Legal Research and Case Analysis
AI models quickly retrieve and analyze vast legal cases and regulations, providing strong support. Legal research platforms using AI can analyze millions of cases in seconds, helping lawyers find relevant precedents and statutes.
Intelligent Contract Review
AI models automatically review contract terms to identify legal risks. Companies use AI tools to find unfavorable clauses in contracts, making timely revisions to avoid disputes.
Legal Education and Training
AI models aid legal education by generating simulated court scenarios and case analyses, enhancing practical skills for students and professionals.
Client Consultation and Support
AI models provide legal consultation through natural language processing, answering client queries rapidly. Legal firms use AI chatbots for 24-hour basic legal advice, improving service efficiency.
Risk Prediction and Compliance Management
AI models assist in predicting legal risks and managing compliance by analyzing operational data and legal environments. They offer strategies to avoid disputes. Multinational companies use AI to monitor global regulations, adjusting strategies to ensure compliance.
III. Education Field
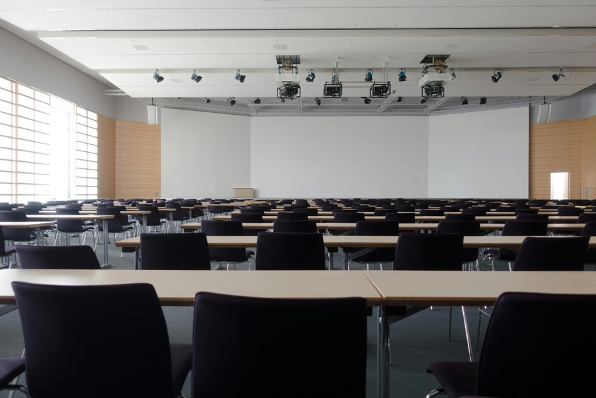
Personalized Learning Paths
AI models create personalized learning paths based on students’ performance and interests. Online platforms analyze learning data to recommend suitable resources and courses for efficient learning.
Intelligent Tutoring and Assessment
AI provides real-time, personalized tutoring and feedback, helping students understand and master knowledge. Math tutoring apps use AI to guide students through problems, overcoming learning obstacles.
Teacher Assistance and Teaching Optimization
AI assists teachers by analyzing exam results and class performance, identifying weaknesses, and optimizing teaching methods to improve outcomes.
Virtual Learning Environments and Simulated Experiments
AI creates interactive virtual environments and simulations, enhancing engagement. Science platforms offer virtual labs where students conduct experiments, observing processes and boosting skills.
Language Learning and Translation
AI models enhance language learning with dialogue simulations and instant translations. They offer pronunciation corrections and grammar suggestions, improving effectiveness.
Teaching Resource Generation and Management
AI models generate resources like test questions and courseware, reducing teacher workload. They classify and manage resources for easy access.
Learning Behavior Analysis and Early Warning
By analyzing behavior data, AI identifies habits and potential issues, providing timely interventions. Educational institutions monitor online engagement, alerting teachers to guide students at risk of falling behind.
Vocational Education and Skills Training
AI offers customized vocational courses and training programs. Platforms analyze market demands to recommend skills courses, enhancing employability.
Conclusion
Although AI large models are still developing and have limitations, they have demonstrated enormous potential, driving progress and innovation in healthcare, law, finance, education, and more. Despite their imperfections, these technologies already play significant roles across fields. Empowered by AI, individuals experience enhanced efficiency and capabilities, feeling transformed.
The integration of AI with professional fields not only improves efficiency and accuracy but also brings new opportunities and directions. In medicine, AI assists in precise diagnoses and treatments; in law, it processes extensive documents swiftly; in education, it offers personalized learning and intelligent tutoring.
As AI technology continues to evolve and proliferate, large models will impact more areas profoundly. Through ongoing optimization, they will foster innovation and transformation across professional domains, benefiting society. We can anticipate deeper integration of AI with specialized fields and widespread personal applications, promoting comprehensive advancement for individuals and industries alike.