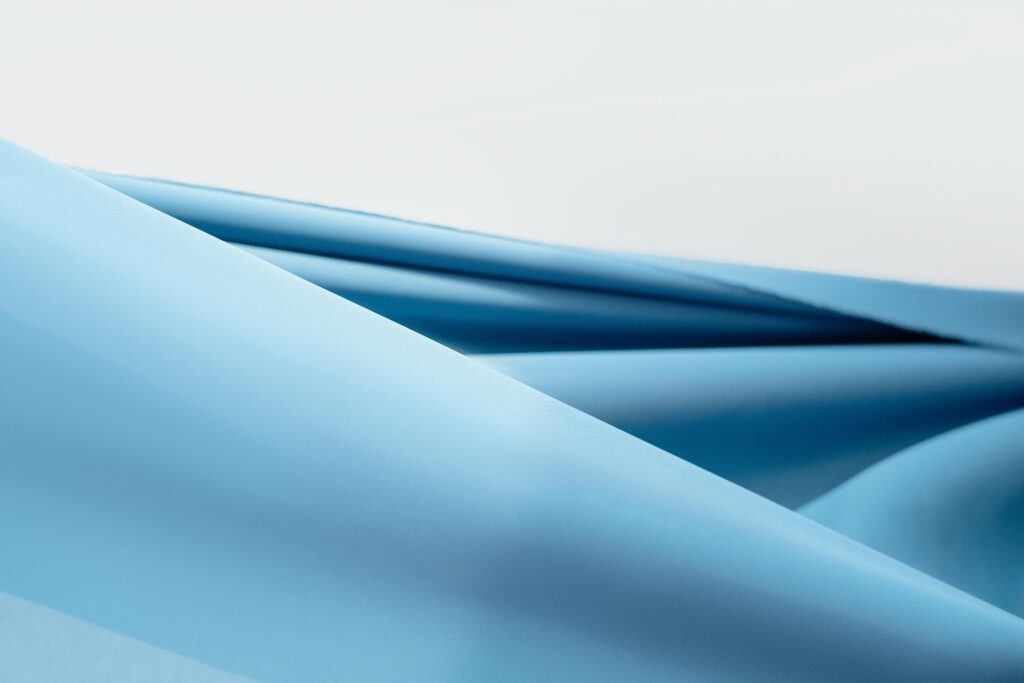
AlphaFold 3 (AF3), developed by Google DeepMind, represents a major advancement in the field of biomolecular structure prediction. It is a state-of-the-art AI tool specifically designed to predict and analyze the three-dimensional structures of complex biomolecules and their interactions, encompassing proteins, DNA, RNA, and other biomolecules. AF3 transcends the limitations of previous versions by employing advanced machine learning techniques to accurately predict the 3D structures of various biomolecules, including proteins, DNA, and RNA, along with their complex interactions. AF3 is capable of handling intricate assemblies of macromolecules and small molecules, elucidating key interactions within multi-molecular systems. These features make it a powerful tool with enormous potential across biological, medical, and pharmaceutical applications. This article provides a comprehensive overview of its key functionalities, capabilities, and practical guidelines for its use in various fields.
Comprehensive Biomolecular Structure Prediction
AlphaFold 3 can predict the structure and interactions of a wide range of biological molecules with unprecedented accuracy [1][4]. Unlike its predecessor AlphaFold 2, which was limited to protein structure prediction, AF3 can model:
- Proteins
- DNA
- RNA
- Small molecules (ligands)
- Ions
- Chemical modifications
This comprehensive approach enables AF3 to generate joint 3D structures of complex molecular assemblies, revealing how different components fit together [1][4].
Advanced Architecture
The enhanced capabilities of AlphaFold 3 are driven by its next-generation architecture:
- An upgraded Evoformer module, which was the core of AlphaFold 2
- A new diffusion network for assembling predictions, similar to those used in AI image generation networks
- A diffusion-based deep learning architecture that directly predicts atomic coordinates [2][4]
This architecture allows AF3 to model a diverse array of biomolecular interactions without the need for excessive specialization for different molecular components [2].
Improved Prediction Accuracy
AlphaFold 3 shows significant improvements in prediction accuracy:
- At least a 50% improvement in predicting interactions between proteins and other molecular types compared to existing methods [1]
- 50% higher accuracy than the best traditional methods in predicting drug-like interactions in the PoseBusters benchmark [1][4]
- Superior accuracy in predicting protein-ligand interactions compared to state-of-the-art docking tools [2]
Applications in Drug Discovery
AF3’s capabilities have significant implications for drug discovery:
- Accurate prediction of interactions involving drug-like molecules, including protein-ligand and antibody-protein binding [1][4]
- Potential to streamline the drug design process by providing insights into protein-ligand interactions [2]
- Already being used by Isomorphic Labs to tackle real-world drug design challenges [1][4]
Accessibility for Researchers
To benefit a wider range of researchers, Google DeepMind has made AlphaFold 3 accessible through:
- AlphaFold Server: A free, user-friendly platform for non-commercial research [1][4]
- GitHub Release: Code and model parameters are available for non-commercial use [5]
This accessibility allows scientists to harness the power of AF3 for a variety of research applications, accelerating scientific discoveries in structural biology and related fields.
Practical Application Guidelines in Biology, Medicine, and Pharmacology
AlphaFold 3 serves as a versatile biomolecular modeling tool that accelerates research in structural biology while also finding applications in medicine and pharmacology. The following are practical recommendations for various research institutions on how to effectively use AlphaFold 3:
1. Recommendations for Biological Research Institutes and University Laboratories
- Analyzing the Complexity of Known and Unknown Molecular Assemblies: Universities and research institutions can use AF3’s predictive capabilities in combination with high-throughput sequencing (NGS), microarray, and other high-throughput laboratory data to delve deeply into the spatial structures of complex molecular assemblies, revealing interactions among proteins, DNA, RNA, and small molecules. For instance, RNAseq or gene expression microarray data can be used to study gene-gene interactions. By integrating these data with AF3 predictions, researchers can uncover more meaningful findings from multiple dimensions, revealing crucial mechanisms in gene regulatory networks.
- Multi-Omics Integration Analysis: In addition to RNAseq and gene expression data, researchers can integrate proteomics, metabolomics, and epigenetics data with AF3’s structural predictions. Such multi-omics data analysis can help elucidate changes in biomolecular interactions under different biological conditions (e.g., disease states or environmental stress), identify key regulatory nodes, and discover potential drug targets.
- Reconstruction of Protein Interaction Networks: AF3 can be combined with experimental data (such as immunoprecipitation and mass spectrometry data) to reconstruct complex protein interaction networks. By predicting the binding patterns between proteins, researchers can better understand how proteins form dynamic complexes within cells. This type of network analysis is crucial for understanding cellular signaling, metabolic regulation, and disease mechanisms.
- Functional Genomics Coupled with Structural Biology: Using CRISPR screening to identify genes critical to specific cellular functions, and then applying AF3 to predict the structure of gene products, researchers can better understand how gene mutations impact protein function and consequently affect cellular processes. This is particularly important for developing precise therapeutic strategies targeting these critical proteins.
- Teaching and Research Applications with AF3: University educators can use AlphaFold 3 in teaching, instructing students on how to use AI tools to analyze the complexity of biomolecular interactions and design experiments to validate these interactions. Laboratories can use AF3 predictions as a foundation, combining them with other molecular simulation tools such as molecular dynamics (MD) simulations to further study biomolecular dynamics under various environmental conditions.
2. Applications in the Medical Field
- Precision Medicine and Personalized Treatment: Medical research institutions can leverage AF3 predictions to design personalized treatment plans. Beyond predicting 3D structures of mutated proteins in patients, AF3 can be used to predict protein-ligand interactions, aiding in the selection of the most suitable drugs. Furthermore, AF3 can analyze key proteins in multiple signaling pathways and predict their structural changes under disease conditions, thus identifying optimal therapeutic targets. For example, in cancer treatment, AF3 can predict the structure of tumor-related mutated proteins to design more effective targeted drugs to inhibit tumor growth. Additionally, AF3 can be combined with high-throughput genomic data to assess how gene expression changes impact protein structure and function, thereby facilitating more precise therapeutic strategies.
- Early Diagnosis and Prediction of Complex Diseases: AF3 also shows great potential in the early diagnosis and prediction of complex diseases. For example, in studies of conditions such as sudden cardiac death, AF3 can predict structural changes in proteins associated with the disease, and, when combined with RNAseq and gene expression microarray data, can identify molecular markers linked to early warnings. This integrated approach helps healthcare institutions better predict disease risks in high-risk individuals, enabling timely interventions and reducing the likelihood of sudden disease events. Moreover, for complex polygenic hereditary diseases, AF3 can assist in identifying how mutations in multiple genes collectively impact protein interaction networks, providing insights for developing multi-target treatment strategies.
- Familial Risk Assessment of Genetic Disorders: By integrating AF3’s protein structure predictions with family genome data, researchers can better assess the transmission risk of hereditary diseases within families. AF3 provides structural insights into protein mutations associated with specific genetic disorders, helping to determine their potential impact on function, and enabling more accurate health risk assessments for family members.
- Personalized Immunotherapy: In immunotherapy, AF3 can be used to predict the binding affinity of tumor-specific antigens (neoantigens) with a patient’s specific major histocompatibility complex (MHC), helping to identify the most immunogenic targets and optimize personalized vaccine design. This application is particularly relevant for personalized cancer immunotherapy, as it can enhance the effectiveness and specificity of immune responses while minimizing side effects.
- Understanding and Treating Rare Diseases: For rare diseases, AF3’s high-precision predictions can help researchers understand the molecular basis of these conditions and design specific small-molecule drugs. Gene therapy companies can use these predictions to design more effective gene editing tools (e.g., CRISPR/Cas systems) to correct pathogenic mutations and improve treatment success rates.
3. Strategies for Use in Drug Development
- Identification and Validation of Drug Targets: Drug development teams can use AF3 to rapidly screen and validate potential drug targets, particularly those that are difficult to identify using traditional experimental methods. Using the precise protein structures generated by AF3, researchers can more efficiently identify key binding sites and perform virtual compound screening on these sites.
- Ligand Docking and Drug Optimization: By integrating AF3 with existing drug screening and molecular docking tools like AutoDock, medicinal chemists can efficiently identify small-molecule candidates with strong binding affinity to targets and validate their activity through experimentation. Additionally, deep learning tools can be used to simulate interactions between drug molecules and targets, improving the affinity and specificity of drug designs.
The Significance of AlphaFold 3’s Open Source Release
1. Transforming Research Paradigms and Broader Applications
Traditional biomolecular structure research relied on time-consuming and costly experimental techniques, such as X-ray crystallography and nuclear magnetic resonance (NMR). AlphaFold 3 has drastically changed this paradigm by shifting research from an experiment-driven to a computation-driven model, significantly increasing efficiency and lowering barriers to entry. For small laboratories and resource-limited research institutions, AF3 provides unprecedented opportunities to participate in high-level structural biology research.
2. Promoting Data Sharing and Global Scientific Collaboration
AF3’s open source policy and integration into public databases have greatly promoted data sharing and international collaboration in research. Scientists can easily access these predictive data, avoiding redundancy while fostering rapid dissemination and accumulation of knowledge. This open collaboration model not only drives innovation in the life sciences but also facilitates interdisciplinary integration, such as with ecology and agricultural sciences, exploring how environmental changes affect molecular functions in organisms, thus enhancing agricultural productivity and ecological conservation.
3. Providing Infrastructure for Future Research
AlphaFold 3 is more than just a tool; it is becoming an essential infrastructure for future scientific research. With improvements in prediction accuracy and the expansion of data scales, AF3 is poised to integrate with other cutting-edge technologies, such as gene editing and synthetic biology, further advancing these fields. It enables scientists to more precisely manipulate biological systems, develop novel drugs and biomaterials, and propel the biotech and pharmaceutical industries into a new era of innovation.
Conclusion
The open-source release of AlphaFold 3 marks a new epoch in life sciences research. It accelerates every stage from basic research to drug discovery, while also offering a new way of understanding biomolecular interactions. Looking ahead, as more technologies are integrated with AlphaFold 3, we anticipate breakthroughs in biology, medicine, and pharmacology that will significantly advance our understanding of life’s mysteries and improve human health. The open-source philosophy of AF3 embodies the ideal that technological progress should benefit all humanity, paving the way for collaborative success and knowledge sharing in the scientific community.
References:
[3] https://www.science.org/content/blog-post/alphafold-3-debuts
[4] https://blog.google/technology/ai/google-deepmind-isomorphic-alphafold-3-ai-model/
[5] https://the-decoder.com/google-deepmind-releases-alphafold-3-for-scientific-use/